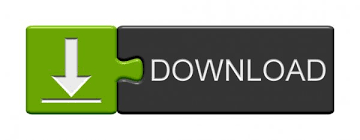
For example, one feature captured how our mouths move when speaking. The team next used these data to train AI models, based on five language features, that can predict how their brains fire up. Alexander Huth at the University of Texas, volunteers listened to hours of podcasts while getting their brains scanned with fMRI. Since its release, the AI and its successors have written extraordinary human-like poetry, essays, songs, and computer code, generating works that stump judges tasked with determining machine from human. Briefly, GPT-3 works by predicting the next word in a sequence. When it comes to language processing, neuroscientists are in the dark.Įnter GPT-3, a deep learning model with crazy language writing abilities. Dubbed the “little brain,” the cerebellum is usually known for its role in motion and balance. But studies are now pointing to a new, surprising hub: the cerebellum.
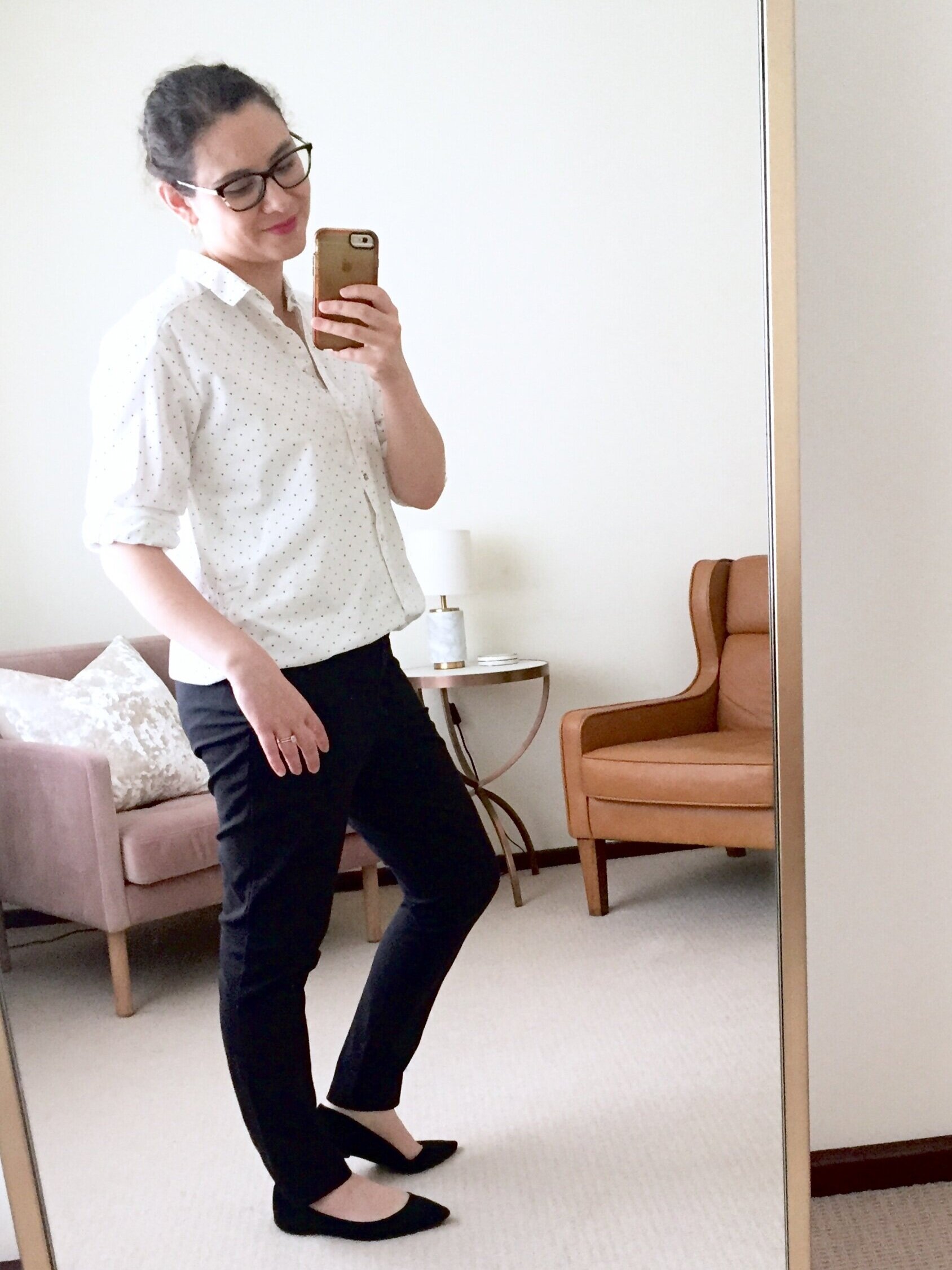
We often think of the cortex as the central processing unit for deciphering language. The ‘Little Brain’s’ Role in LanguageĪs an example, Fyshe turned to a recent study about the neuroscience of language. One thing is clear: when it comes to using AI to inspire neuroscience, “the future is already here,” said Fyshe. Similarly, several computational strategies the brain uses aren’t yet used by deep learning. Incorporating molecular data into artificial neural networks could nudge AI closer to a biological brain, he argued. Although we often talk about the brain as a biological computer, it runs on both electrical and chemical information. Cian O’Donnell at Ulster University, the answer is a lot. “AI have already been useful for understanding the brain…even though they are not faithful models of physiology.” The key point, she said, is that they can provide representations-that is, an overall mathematical view of how neurons assemble into circuits to drive cognition, memory, and behavior.īut what, if anything, are deep learning models missing? To panelist Dr. Alona Fyshe at the University of Alberta agrees. Discovering what to include-or exclude-is an enormously powerful way to find out what’s critical and what’s evolutionary junk for our neural networks.ĭr. Sara Solla, an expert in computational neuroscience at Northwestern University’s Feinberg School of Medicine. “It’s not wrong to use simplified models,” said panel speaker Dr.
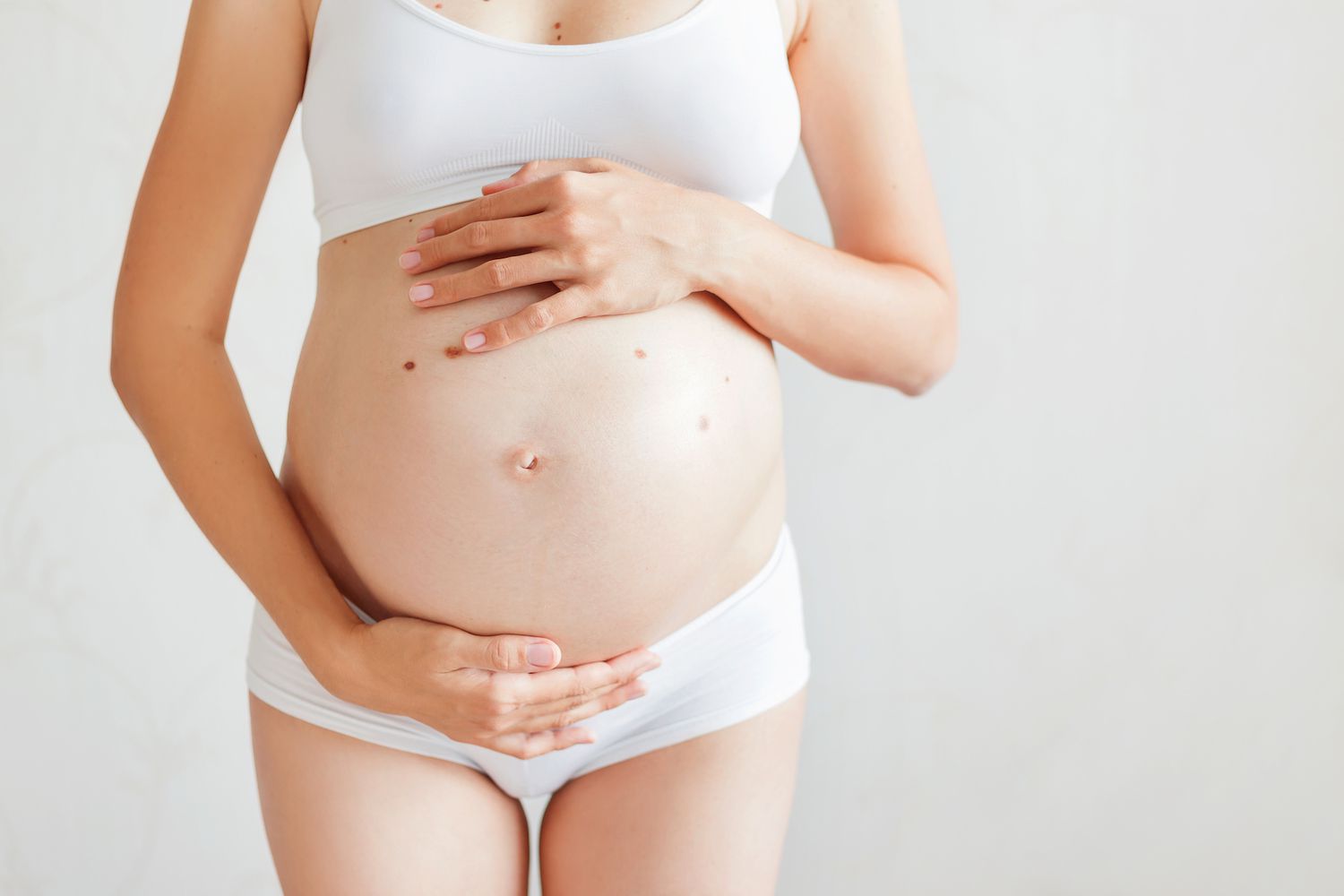
By studying how deep learning algorithms perform, we can distill high-level theories for the brain’s processes-inspirations to be further tested in the lab. But that’s not the point, argues the panel. In fact, it contains elements that are biologically improbable, if not utterly impossible. Deep learning wasn’t meant to model the brain.
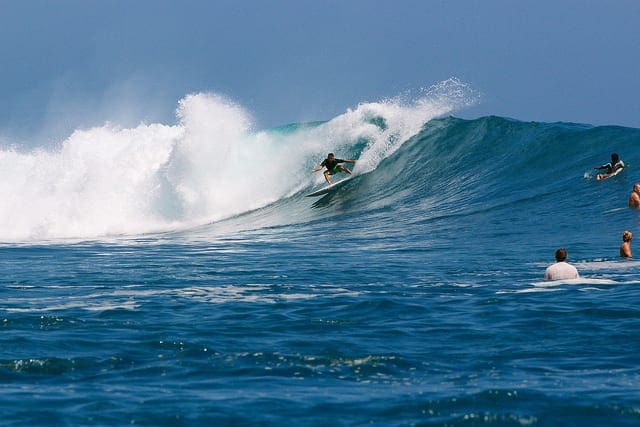
Yet compared to human brains, these algorithms are highly simplified, even “cartoonish.”Ĭan they teach us anything about how the brain works?įor a panel at the Society for Neuroscience annual meeting this month, the answer is yes. Artificial neural networks are famously inspired by their biological counterparts.
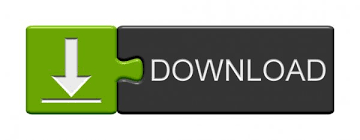